Background
In recent years, advanced analytical and artificial intelligence (AI) capabilities have become commoditized. Organizations from all sectors are exploring ways in which they can leverage these capabilities for their business. The increased demand for analytics and proliferation of lower cost sensors and the Internet of Things (IoT) has enabled more data collection. Also supported further by advances in processing and networking capabilities, the increased demand has led to increased adoption of analytics.
These technological advances have paved the way for “as a service” applications in the cloud. As a result, many companies have been able to reduce the barrier to entry into the field of analytics. Companies working with real-time data in industries like oil and gas are also seeking ways to leverage these new capabilities. In this article, we take a step back and discuss general approaches to analytics, the types of applications available in this field, example use cases, and the general roadmap to digital maturity.
General Approaches to Analytics
Although the hype in recent years has been about AI and machine learning, there are several different ways in which to approach data analytics. Broadly, the data-driven analysis techniques fall into two categories – data-driven and principles-driven.
Data-Driven
Data-driven analytics leverage mathematics and data processing to produce insights. They may draw upon engineering principles to inform certain aspects, such as input feature weighting. However, they largely rely on machines drawing conclusions without humans telling them how. There are two high-level sub-types within this category:
- Mathematical Models – deterministic mathematical techniques such as calculus, regression, or statistical aggregates to identify anomalies and make predictions.
- Advanced Analytics – non-deterministic mathematical and statistical techniques and includes machine learning and AI for pattern recognition.
Principles-Driven
Principles-driven analytics are guided by scientific laws or empirical analysis. The methodology to obtain an analysis output is determinate. There are two broad sub-types:
- First-Principles – based on thermodynamic and physical laws. For example, calculating pump or heater efficiency by examining the ambient process variable data including temperatures, flows, pressures, etc.
- Rules-Based – based on observations and empirical analysis. Often these are conceptualized in the form of fault trees, leveraging Failure Mode Effect Analysis (FMEA). Failures are broken down into root cause failures. A combination of different root cause failures may suggest a high probability of impeding failure modes at the top levels of the fault tree.
Types of Applications
There is a plethora of toolsets in the analytics space, especially as it relates to operational data and this is continually evolving.
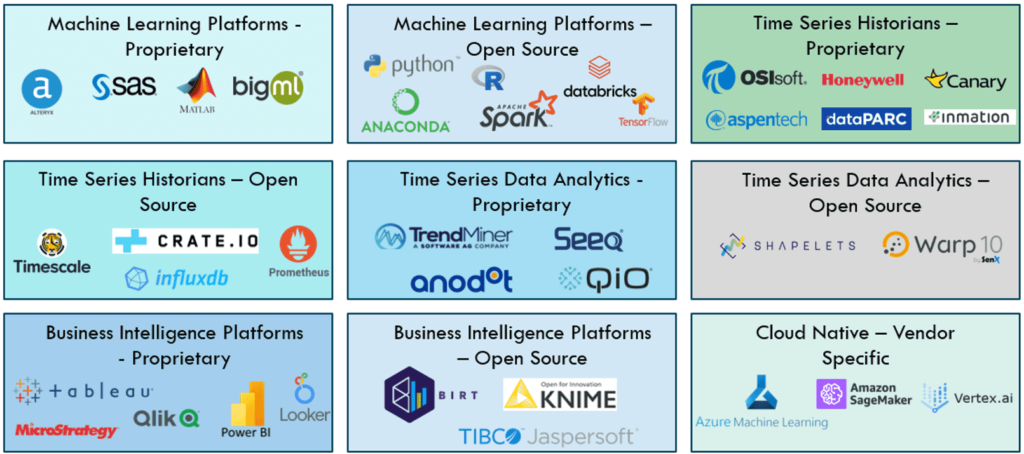
Time Series Historians – whether proprietary, open-source, or cloud-native, have analytical capabilities that will enable users to configure and execute calculations. Calculations implemented here are largely deterministic and cater to most principles-driven analytics and mathematical models. Time-series historian toolsets are often constrained by their AI or advanced analytical capabilities.
Time Series Data Analytics – specialized toolsets meant to run statistical analysis, pattern recognition, or other mathematical calculations on time series data. These toolsets are often conducive to ad-hoc analysis. However, they are not typically geared toward real-time integration in an operational capacity. It is often difficult to tie back analytical results into the operational data infrastructure.
Business Intelligence Platforms – a broad category of tools that contain data aggregation, transformation, modeling, and reporting capabilities. Some tools allow for abstract modeling and most support multi-dimensional aggregation. Companies often use BI tools to combine operational data with data from other departments. Business intelligence toolsets provide aggregate views often in an ad-hoc capacity (over online transaction processing – or OLTP) over long time periods. Data analysis is more descriptive in nature and does not focus on predictions. Companies do not use the data for real-time consumption since the data is often older and aggregated.
Machine Learning Platforms – varied and can include cloud-native services or localized installations of tools and technologies. Machine learning platforms focus more on the advanced mathematical analysis side and often require data integration to enable learning and feedback. Many companies find that heavy data engineering is needed to curate the data in the formats that required for machine learning.
Example Use Cases
Analytics can be broadly applied to any example or use case where there is sufficient good quality data and an understanding of the process. In the table below, we have listed the most common applications of analytics using the listed analytical approaches that we at MetaFactor have observed. Some use cases can be handled with more than one approach. Note that these are just a few use cases and within each business, there are nearly endless possibilities.
Data-Driven Analytics | Principles-Driven Analytics |
Mathematical Models Leak detection Anomaly detection Rate and volumetric totalizers Capacity utilization Hydrocarbon accounting Advanced Analytics Pump failure prediction (e.g., PCP, ESP) Rock strength analysis Heat exchanger fouling Corrosion detection Wellbore stability prediction Drilling optimization | First-Principles Pump efficiency Heat exchanger efficiency Compressor efficiency Equation of state Turbine compression ratio Rules-Based Pump failure prediction (e.g., PCP, ESP) Motor failure Poor system performance Asset performance management |
Roadmap to Digital Maturity
We at MetaFactor have helped several organizations through their digital transformation journey. We have observed the following common pattern for companies that want to become more digitally mature.
Establish Digital Foundations
The first step is always making sure you have a solid foundational data infrastructure in place. Often, this involves an initial audit of the system and as-is architecture to understand and address shortcomings. Once a right-sized architecture is in place, then it is time to setup real-time data collection. Good quality data means that the data must be consistent, reliable, and frequent enough for analytics. Companies should also pay attention to metadata and make sure that units of measure, descriptions, and other parameters are accurate. Once this is complete, end users must be trained as well. This ensures a solid and trustworthy data foundation.
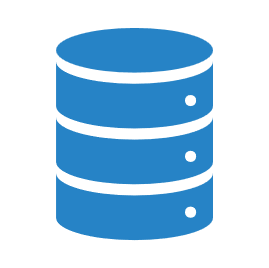
Targeted Use Cases
Next, companies should identify targeted use cases – ideally simple ones with a strong feasibility. Companies may choose to select one or more immediate problems or optimizations that are realistic. Early success here is important since it will help users build overall confidence within the system to support data analytics. Companies will build and refine models and calculations and iterate until a successful outcome is achieved. Once complete, it is best to capture lessons learned.
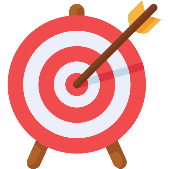
Scale Up
The next phase involves scaling up. Where applicable, the initial pilot use cases should be expanded out fleet wide. At the same time, this phase should include some thought around definition of standards and governance. Before growing their analytics practice too far, companies will want to standardize on many fronts. This includes the sources and egress points for data analytics, revisiting tools and technologies used, security, determining the tiering of different environments, testing requirements, and so on. In general, businesses should adopt a philosophy of continuous improvement in this area.
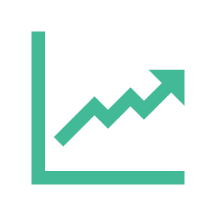
Digital Leadership
Finally, we arrive at the digital leadership stage. Leading organizations continually leverage technology to achieve strategic objectives. There is pervasive data-driven decision making across the organization. These organizations also stay abreast of technological developments and continuously evolve and adapt. This may mean that there are dedicated C-suite roles setup to drive digital transformation and a culture of innovation. Like in any stage, there should be an emphasis on continuous improvement.
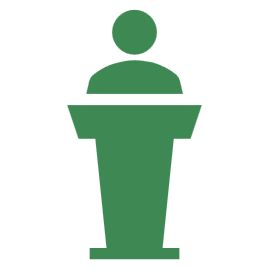
We Can Help
We are specialists in historian systems, analytical and cloud toolsets, and reporting and business intelligence tools that can help your organization achieve these benefits. The MetaFactor team are Calgary OSIsoft PI Experts and Calgary OSIsoft AF Experts. Talk to us today to find out how we can help your company.